Data Ethics, Relational Analytics, and Leading Successful Data Science Teams
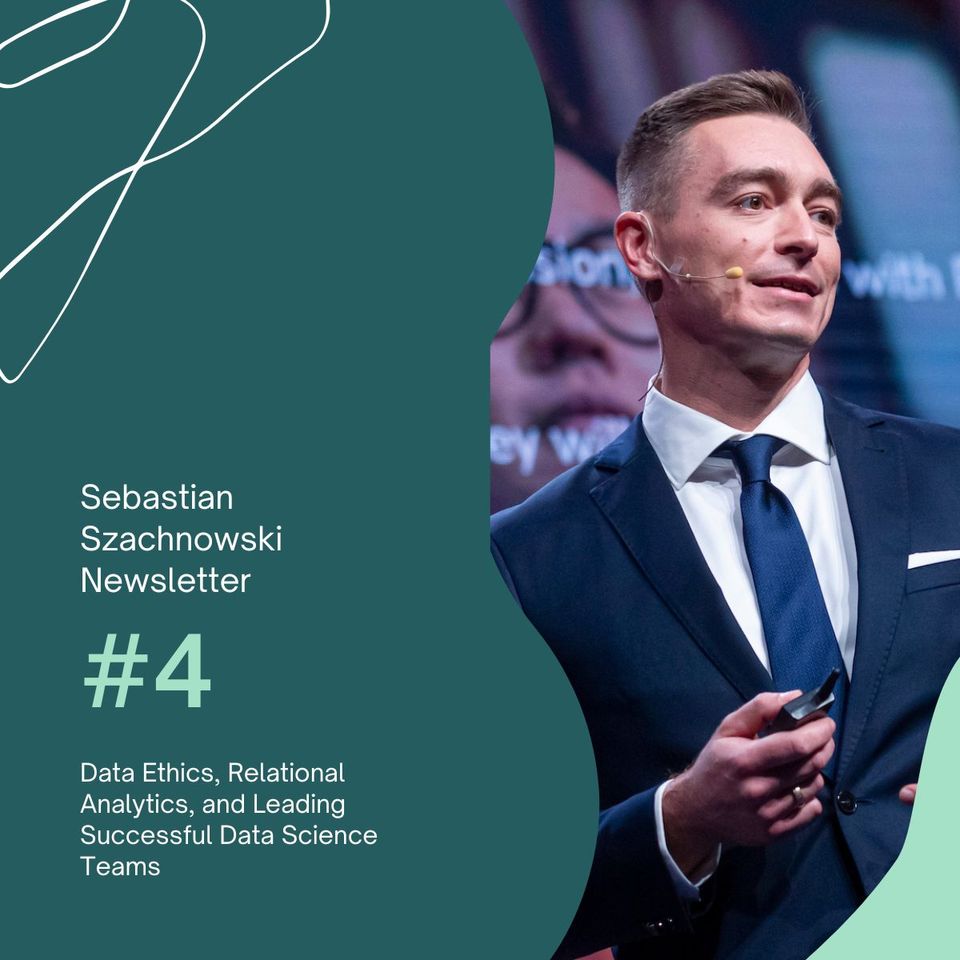
Hi!
Data ethics is key in people analytics not only because there is a strong regulatory about data privacy and people sensitive information, but because trust and transparency in PA team’s actions will preserve trust of employees who will be more likely to give their consent to process their data. Besides, ethical stance is the value that we owe to every human being. Period.
In this edition of my newsletter, we deep dive into ethics in data management. Then, we will turn into Relational Analytics, which is also known as Organization Network Analysis (ONA), and Social Network Analysis (SNA). A very powerful tool in right hands. I’m a big proponent of it, however, it requires trust and high ethical standards, as it assumes using passive digital data from the collaboration tools used at the workplace. In the end, we will identify the guidelines to lead successful data science team and – as a bonus – I’ll show you how to get for free a highly rated book about data science.
Table of contents:
- Data Ethics
- Relational Analytics
- Guideline to Lead Successful Data Science Team
- Interesting Articles
- Free e-book!
I hope you will enjoy this issue of my newsletter!
Data Ethics
Data ethics could be defined as data-related practices that seek to preserve the trust of users, patients, consumers, clients, employees, and partners. The characteristics of ethical data use are:
- It preserves data security and protects customer information
- It offers a clear benefit to both consumers and companies
- It offers customers some measure of agency
- It is in line with your company’s promises
By 2025, individuals and companies around the world will produce an estimated 463 exabytes of data each day, compared with less than three exabytes a decade ago.
Companies should address the ethical standards of data management which could have complex and unwelcome consequences and responsibilities. Mishandled algorithms might incur significant reputational and financial costs to companies.
Potential challenges regarding the data ethics include thinking that data ethics doesn’t apply to your organization. Privacy and ethical considerations often aren’t top of mind of company executives. Sometimes business leaders are unintentionally pushing this subject away. It’s easy to focus on tangible things like tools, technologies, and strategic objectives associated with data management than on such intangibilities as data ethics.
Another challenge is thinking in silos and assuming that legal, compliance, or data scientists have data ethics covered. The data ethics is everyone’s responsibility, not just the province of data scientists or legal and compliance teams. Employees across the organization will have to raise, act, and think through various ethical issues in surrounding data. Business leaders must ensure that their strategic and commercial objectives are in line with customers’ expectations and with regulatory requirements for the data handling.
Another mistake regarding data ethics is chasing short-term ROI. Executives and employees might be tempted to make unethical data choices to chase short-term profits. At one hand companies set high standards for the use of data, but at the other hand the short-term financial pressures remain.
It’s tempting to collect as much data as possible and to use as much data as possible. Because at the end of the day, my board cares about whether I deliver growth and EBITDA… If my chief marketing officer can’t target users to create an efficient customer acquisition channel, he will likely get fired at some point—or at least he won’t make his bonus.
Another challenge for data ethics is when we’re looking only at the data, not at the sources. Companies must consider the entire data pipeline. Diligence is key.
Where did the data come from? Can this vendor ensure that the subjects of the data gave their informed consent for use by third parties? Do any of the market data contain material non-public information?
Above mentioned data management challenges are common, and they are by no means the only ones. As companies progress with data analytics development, new privacy and ethical challenges will inevitably emerge. Below seven data-related principles may be useful to build fault-tolerant data management.
Set company-specific rules for data usage. Such data usage framework should reflect a shared vision and mission for the company’s use of data. The leadership team should define data rules that give employees a clear sense of the company’s threshold for risk and which data-related ventures are OK to pursue and which are not.
Leaders must come together to create a data usage framework that reflects a shared vision and mission for the company’s use of data.
Data usage rules should be accessible to all employees, partners, and other stakeholders. Business leaders should revise these rules periodically to account for shifts in the business and technology.
Communicate your data values, both inside and outside your organization. Once common data usage rules are stablished, it’s important to communicate them effectively to all interested parties. It might be tailored discussions about data ethics with various business units and functions. The message to IT and data scientists might be about creating ethical data algorithms. The message to marketing and sales might be about transparency and opt-in/opt-out protocols. The message to HR might be about using the data only to benefit the employees.
Build a diverse data-focused team. Some companies have appointed chief ethics or chief trust officers. Others have set up interdisciplinary teams, sometimes referred to as data ethics boards, to define and uphold data ethics. Ideally, those boards would include representatives from multiple various functions.
An organization will be more likely to identify issues early on (in algorithm-training data, for example) when people with a range of different backgrounds and experiences sit around the table.
Engage the champions in the C-suite. It does not mean involving CEO directly in the decision-making process, but rather bringing all data ethics conclusions to him and make sure that he agrees with the stance. Having a champion or two in the C-suite will signal the importance of data ethics to the rest of the organization and support the case of investment in data-related initiatives.
Consider the impact of your algorithms and overall data use. Companies should constantly assess and test for bias throughout the value chain. Certain data applications require far greater scrutiny and consideration. It’s important to consider not only what types of data are being used but also what they are being used for.
Think globally. Company leaders must take high level view of their companies as players in the digital economy, the data ecosystem, and societies. They might support policy initiatives or otherwise help to bridge the digital divide, support the expansion of broadband infrastructure, and create pathways for diversity in the tech industry.
Embed your data principles in your operations. Data ethics boards, business unit leaders, and C-suite champions should build a common view and language about how data usage rules should connect to company’s data and strategies and to real use cases of data ethics. Leaders should identify KPIs that can be used to monitor performance in realizing data ethics objectives. The leadership team should also advocate, help to build, and facilitate formal training programs on data ethics.
Organizations that fail to walk the walk on data ethics risk losing their customers’ trust and destroying value.
Relational Analytics
Not everyone knows that in talent management people interactions are equally meaningful as statistical insights.
Research shows that a lot of employees’ success can be explained by their relationships—something that’s the focus of a new discipline, relational analytics. The key is finding “structural signatures”: patterns in social networks that predict who will have good ideas, which employees have the most influence (it’s not senior leaders), which teams will be efficient, which will innovate best, where silos exist, and which employees firms can’t afford to lose.
Although, people analytics has become mainstream, only few companies believe they have a good understanding of which talent dimensions drive performance in their organizations. This might be because they use only data on individual people, when data about the relations among people is equally or more important.
Relational analytics helps companies better identify employees who are key to achieve company’s goals, whether for increased innovation, influence, or efficiency. Firms also gain insight into which key employees they can’t afford to lose and where silos exist in their organizations.
By default, people analytics focuses on employee attributes:
- Trait: facts about individuals that don’t change, such as ethnicity, gender, and work history.
- State: facts about individuals that do change, such as age, education level, company tenure, value of received bonuses, commute distance, and days absent.
Attribute analytics is necessary but not sufficient. As relational data captures for instance the communications between two people in different departments in a day, the relational analytics augment people analytics with the science of human social networks. The relationships employees have between them together with their individual attributes can explain the workplace performance.
Structural signatures are patterns in the data that correlate to some form of good or bad performance. Organizational leaders can look at structural signatures in their companies’ social networks and predict creativity or effectiveness of individual employees, teams, or the organization as a whole.
Ideation signature pictures good idea generators who synthesize information from one team with information from another to develop a new product or concept. Sometimes, they use solution created in one division to solve a problem in another. Constraint is a measure that captures how limited a person is when collecting unique information.
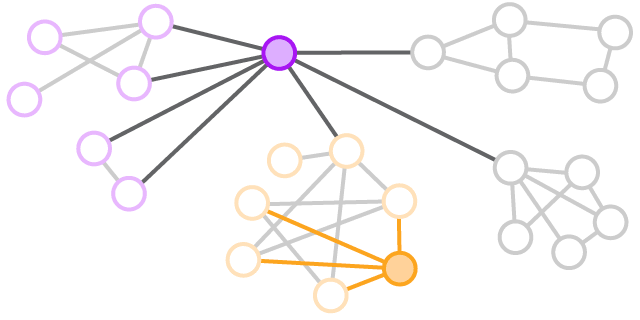
Influence signature pictures which employees will change other people behaviour. The greatest influencers are people who have strong connections to others, even if they connect only to few people. Moreover, their strong connections have strong connections with other people. This way influencers’ ideas spread further. This is called aggregate prominence that is computed by measuring how well a person’s connections are connected, and how well the connections’ connections are connected.
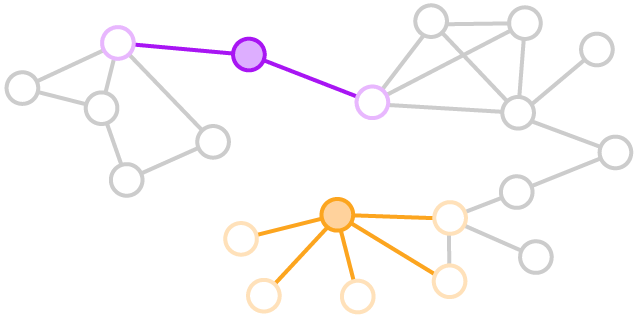
Efficiency signature helps measure team’s chemistry and the ability to draw on outside information and expertise. There are two social variables associated with higher performance. Internal density is the number of interactions and interconnectedness among team members. High internal density helps build trust, taking risks, and reaching agreement on important issues. External range measures the range of team members contacts. The teams with high external range are better able to gather vital information and secure resources they need to meet deadlines. The structural signature for efficient teams is therefore high internal density plus high external range.
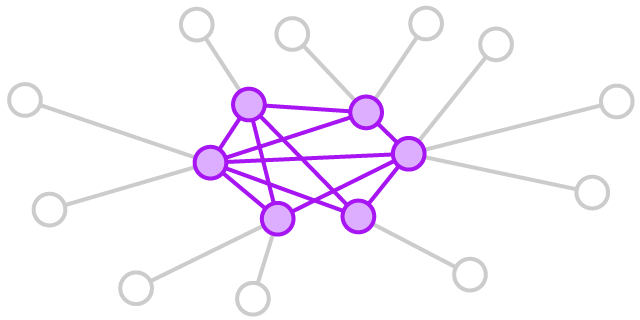
Innovation signature shows which teams will innovate effectively. The same variables we used for team efficiency - internal density and external range – will help identify or build promising innovation teams. The innovation signature is, however, high external range and low internal density. Employees will have wide, non-overlapping social networks to source diverse ideas and information, but they should not be tight-knitted team, as greater interaction with the team results in similar ways of thinking and less discord. The high external range is also needed to garner support and buy-in so they can sell their ideas.
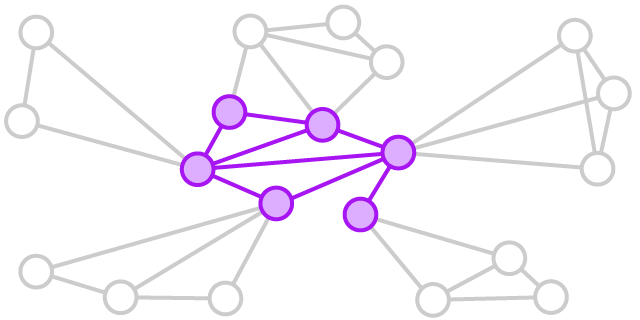
Silo signature is when functions, departments, and divisions become less and less able to work together. They don’t speak the same technical language or have the same goals. Usually, people within the departments are deeply connected, but only one or two people in any department connect with people in other departments. Modularity is the ratio of communication within a group to communication outside the group. When the ratio of internal to external communication is greater than 5:1, the group is siloed.
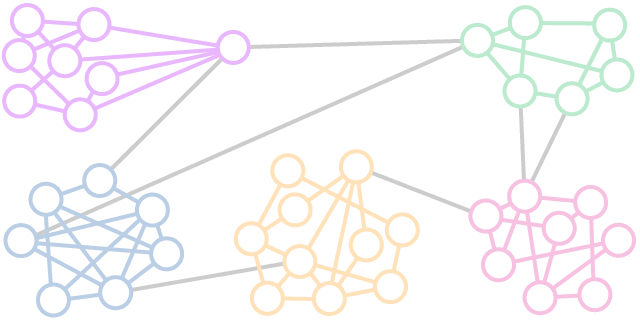
Vulnerability signature identifies which employees the organization can’t afford to lose. Organization relaying on individuals would lack robustness. Networks are robust when connections can be maintained if you remove nodes.
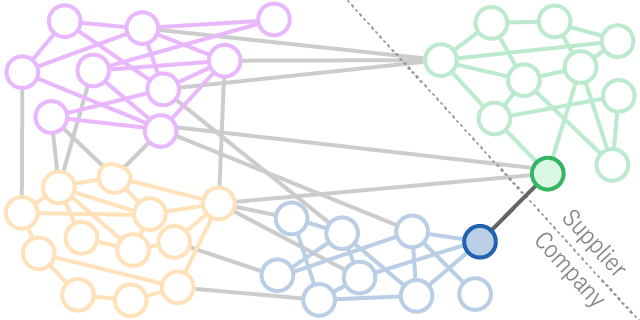
To gather relational data, companies typically survey employees about whom they interact with. However, surveys take time, and the answers can vary in accuracy. All companies have their digital exhaust: the logs, e-trails, and content of everyday digital activity. All platforms record interactions if an employee send an e-mail, message on Slack, post on LinkedIn, form a team in MS Teams, assign people to MS DevOps, etc. This information can be used to create views of employee, team, and organizational networks in which you can pick out the structural signatures we’ve discussed.
Company-collected relational data, however, creates new challenges. Although most employment contracts give firms the right to record and monitor activities conducted on company systems, some employees feel that the passive collection of relational data is an invasion of privacy. This is not a trivial concern. Companies need clear HR policies about the gathering and analysis of digital exhaust that help employees understand and feel comfortable with it.
Also, behaviours aren’t equal. Liking somebody’s post is different from working with somebody in a team for 2 years. Copying somebody on an e-mail doesn’t indicate a strong relationship. All behaviours should be weighted and combined accordingly. ML algorithms and simulation modelling can be helpful.
To be useful, relational data should be timely. Constant updating is required. Also, analyses should be done in close collaboration with the decision makers. Data scientist don’t know the employees they are running analyses on, so that they cannot place results in the right context.
The last but not least, dashboards are key. System that visualizes and highlights identified structural signatures move analytics insights closer to the managers who need them.
Source: https://hbr.org/2018/11/better-people-analytics
Guideline to Lead Successful Data Science Team
Leaders need to guide data teams by clearly identifying problems and setting metrics to gauge success.
Data science teams can be a great source of value for the business. However, they would require proper guidance to succeed:
- Clearly identified problems
- Setting metrics to evaluate success
- Taking a close look at results
Point data science teams toward the right problem. Leaders are responsible to help data science teams focus on the right problem. Depending on the goal, both the path taken by the data science team – including the training data, modelling approach, and level of effort – and the impact on the business, will be different. When comparing with other companies in the industry focus on what they are solving with data science, not on how they are doing this.
Decide on a clear evaluation metric up front. To solve a problem, data science teams typically build lots of models and then select the one that seems best. To make this selection they need a metric. Leaders need to use business judgement to determine what that metric should be. Out of the many models the team will build, what metric will indicate the best one? If you are not sure what metric to use, ask your data science team to educate you on the metrics typically used in the industry to evaluate models for similar problems.
Create a common-sense baseline which is how your team would solve the problem if they didn’t know any data science. For example, a simple baseline is tracking what product categories visitor look at, and recommending best-selling product from those categories. Note, that common-sense baselines are often hard to beat by data science models. Also, when beaten, the data science models might do so just by slim margins.
Manage data science projects more like research than like engineering. In case of data science commitment to clear timeline is a mistake. The fair amount of time in data science is spent on dead ends with nothing to show for the effort. This is trial and error which makes it hard to predict when a breakthrough occurs.
In 2006, Netflix invited data scientists from all over the world to beat their in-house movie recommendation system. The first team to show a 10% improvement would be awarded a $1 million grand prize, and 41,305 teams from 186 countries jumped into the fray. Even so, it took three years for the 10% barrier to be breached.
Leaders can meet regularly with their data science teams to understand the ups and downs of data science work.
Check for ‘truth and consequences.’ It’s important to check the results and intensively control them to make sure the benefits are real and there are no unintended negative consequences. There’s always a need for judgement about the trade-off between one metric and another, and business leaders should be involved in making those decisions. For example, increasing one parameter of sales may have negative consequences for other conversions.
Log everything and retrain periodically. Models can produce unexpected or incurred predictions with certain types of input data. If all inputs are logged, investigating and fixing problems will be easier and faster. As the models over time will start to drift away from the data used to build the model, it is important to make sure that data science teams have automated processes in place to track model performance over time and retrain as necessary.
Data science models, like software in general, tend to require a great deal of future effort because of the need for maintenance and upgrades.
Source: https://mitsloan.mit.edu/ideas-made-to-matter/6-steps-leading-successful-data-science-teams
Interesting articles
- Thoughts On Building a Data & Analytics Practice https://harshitagirase.medium.com/thoughts-on-building-a-data-analytics-practice-4d06bccf9b54
- The Three Cities Problem in People Analytics https://directionallycorrectnews.substack.com/p/the-three-cities-problem-in-people
- Designing XAI for People Data https://towardsdatascience.com/designing-xai-for-people-data-f41bc4cf1fc8
- Do top earners work more hours? It depends which country they live in https://www.weforum.org/agenda/2022/09/working-hours-america-income-economy/
- Research Proves Your Brain Needs Breaks https://www.microsoft.com/en-us/worklab/work-trend-index/brain-research
- How to build an analytics team for impact in an organization https://towardsdatascience.com/how-to-build-an-analytics-team-for-impact-in-an-organization-21bb05925587
Free e-book
Data Science For Dummies 3rd Edition, by Lillian Pierson
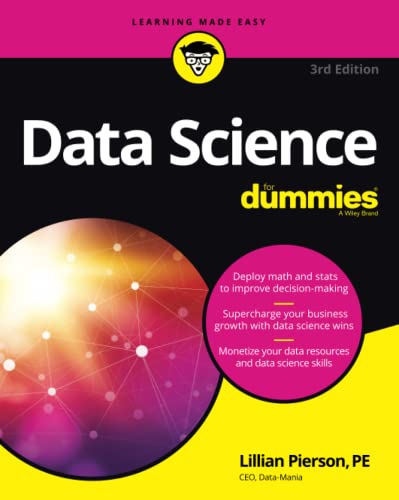
For a limited time, Willey is giving away for free – well, almost for free, as you will have to give them your company email and consent to contact you – a highly rated book (4,7 of 5 on Amazon, based on 45 Reviews).
On its 400+ pages, Data Science For Dummies demonstrates a process to lead profitable data science projects, reverse-engineered data monetization tactics, the truth about how simple natural language processing can be, and how to cultivate your own unique blend of data science expertise.
Regular book price tag is $21.
Download: https://programminglanguage.tradepub.com/free/w_wile392/prgm.cgi
Inspiring quote
“Some of the most successful people I’ve ever known achieved what they did because they built their careers around their strengths, not their weaknesses.” – Jim Clifton
Thank you for reading my newsletter! I’ll be delighted to get your feedback about the newsletter and this issue.
Do you know someone who might be interested in this newsletter? Share it with them.
You can also:
- Subscribe to my newsletter https://szachnowski.com/
- Connect with me on LinkedIn https://www.linkedin.com/in/sebastianszachnowski/
- Follow me on Twitter https://twitter.com/sszachnowski
- Follow me on Instagram https://www.instagram.com/sszachnowski/
- Send me an email sebastian@szachnowski.com