Turnover Contagion, How People Analytics Is Transforming the HR Landscape, and Predictive Retention Projects
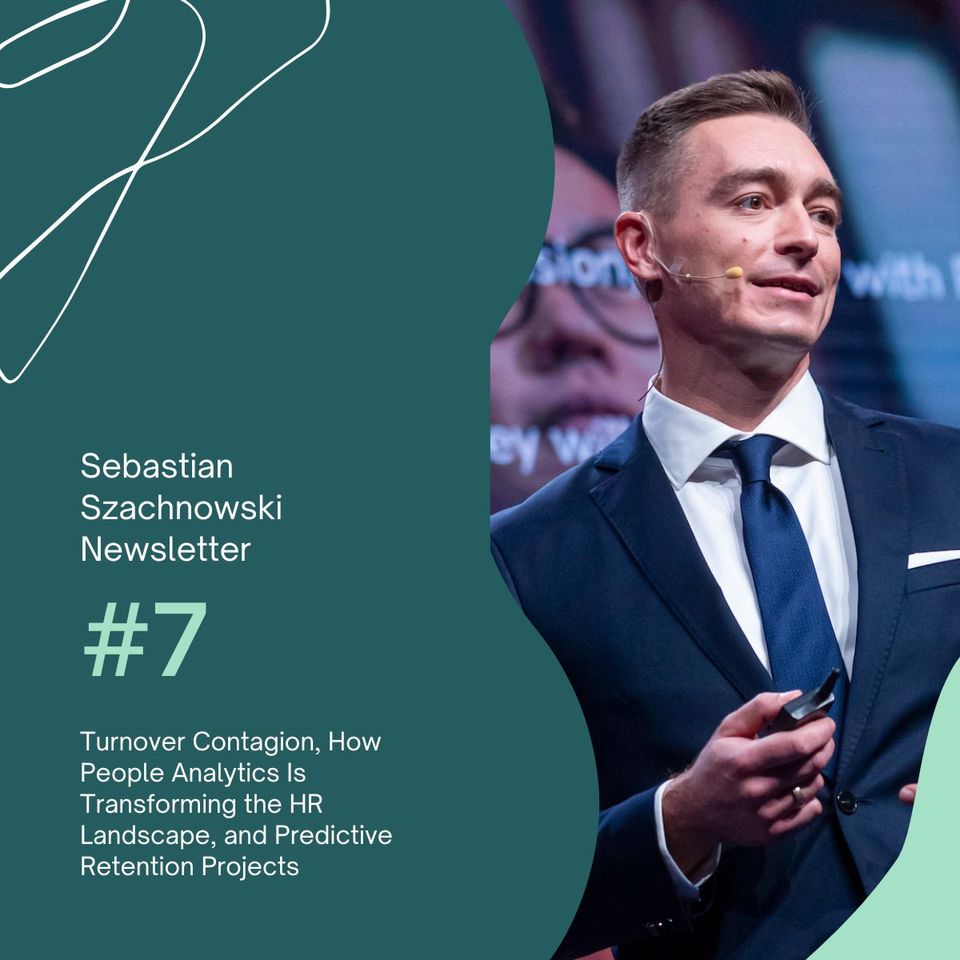
Some time back, Visier has published a report regarding turnover contagion. I believe that every HR professional, leader, entrepreneur, and executive should read it. Within the report, Visier defines, tests, and concludes regarding turnover contagion. The researchers proves that after the first resignation a subsequent leave in the team is 9.1% more likely to happen. The smaller the size of the team is, the stronger effect of turnover contagion. For example, for teams 6-10 people the risk of subsequent leave rises to 14.6%.
Turnover contagion is very important factor to include in turnover predictions, but it also should trigger specific actions from managers to mitigate its impact. Especially in hybrid or remote environment where the bends with the organization, in particular connections outside of the team, are the weakest.
In this issue:
- Turnover Contagion
- How People Analytics Is Transforming the HR Landscape?
- Predictive Retention Projects
- Top 10 Most Demand Skills for the Next 10 Years
- Free E-book About Regression Modelling in People Analytics!
Enjoy!
Turnover Contagion
There are many personal, professional, and economic reasons that somebody leaves a company. However, the phenomenon of employees quitting their jobs because their peers resigned is known as “turnover contagion”.
Many organizations underestimate the impact that one person’s resignation can have on their peer’s decision to leave or stay.
Turnover contagion is defined when employees are transmitting their intention to leave to people around them. It occurs because we tend to compare ourselves to others, or imitate others’ withdrawal from the organization.
Visier has studied that turnover contagion is a real phenomenon that can be detected and measured. To see how severe issue it is, we should consider that direct and indirect costs of voluntary leave have been calculated by Visier to be about $110,000 per leaving employee. However, there are also additional costs to the company, such as:
- Jeopardized organization’s ability to achieve its planned business goals
- Increased the need for external hiring that drives the wages up for both new and rehires
- Disruption and frustration for remaining team members
Many turnover models look at resignations as individual occurrences. However, social psychologists believes that how someone’s colleagues see the world, and how they behave at work matters as people naturally look to others. This includes when making decisions about whether or not to start looking for another job. Employee resignation is not isolated event, but happens in a social setting.
Visier studied if an initial resignation by an employee would lead to more, earlier, and more frequent resignations by their teammates under the same manager, on the same team. For each group on the same team, they created a second “control” group of the same size by randomly assigning employees as long as they were not part of the same team. This allowed to measure the effects of resignation on other team members by contrasting them with employees that are not teammates.
When a resignation occurs, employees on that team are 9.1% more likely to leave within the next 135 days than employees who are not on that team.
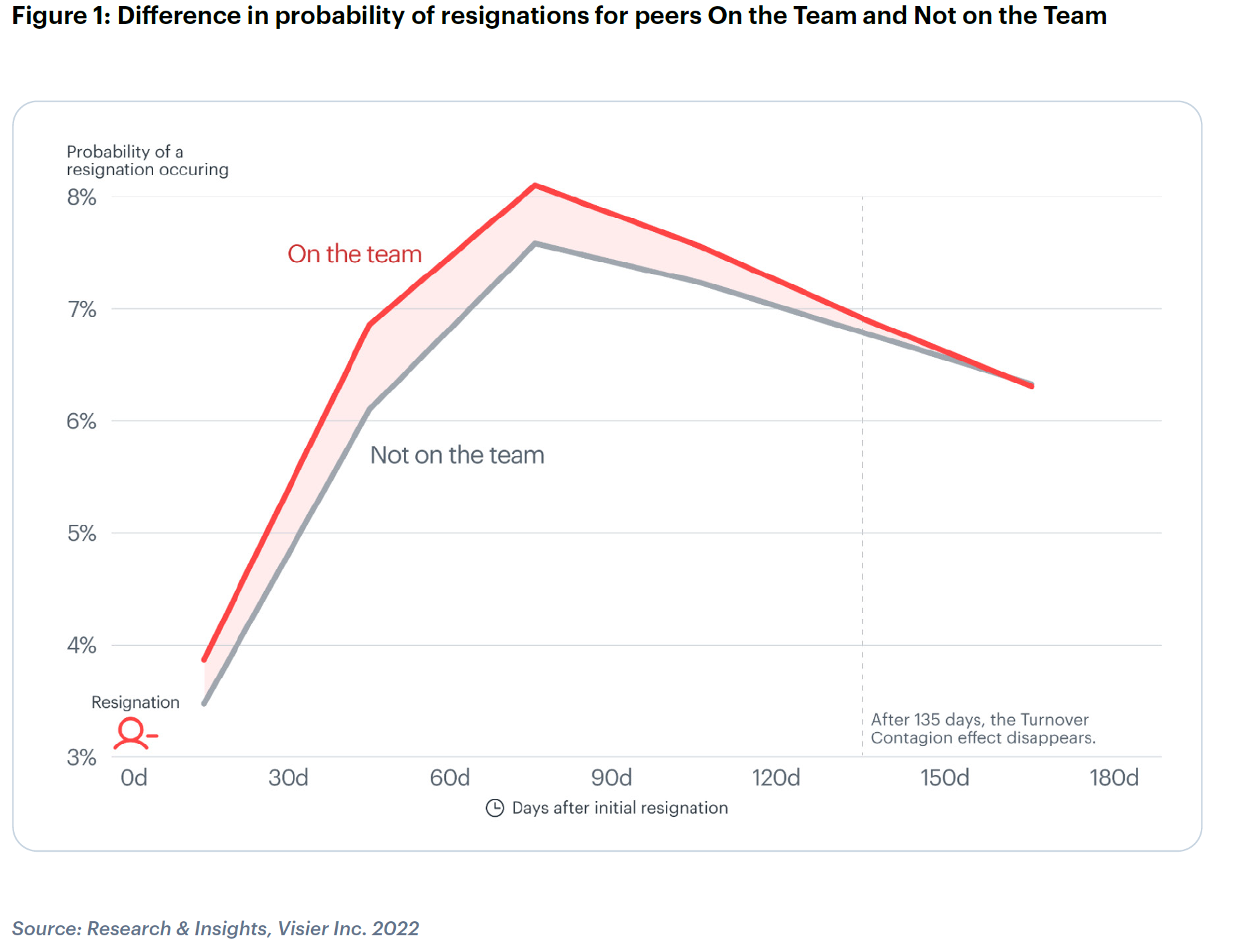
Visier found that after the initial resignation of any employee their peers subsequent resignations increased. Despite the population outside the team also experienced resignations throughout the same time period, the resignations in the team happened earlier and in higher frequency after their first colleagues resigned. This supports the turnover contagion hypothesis. The probability of further resignation is amplified by turnover contagion effect by 9.1%. Subsequent resignations were observed on the period from 0 to 135 days after the initial resignation.
Turnover contagion effect is stronger on smaller teams which have higher probability to experience subsequent resignation.
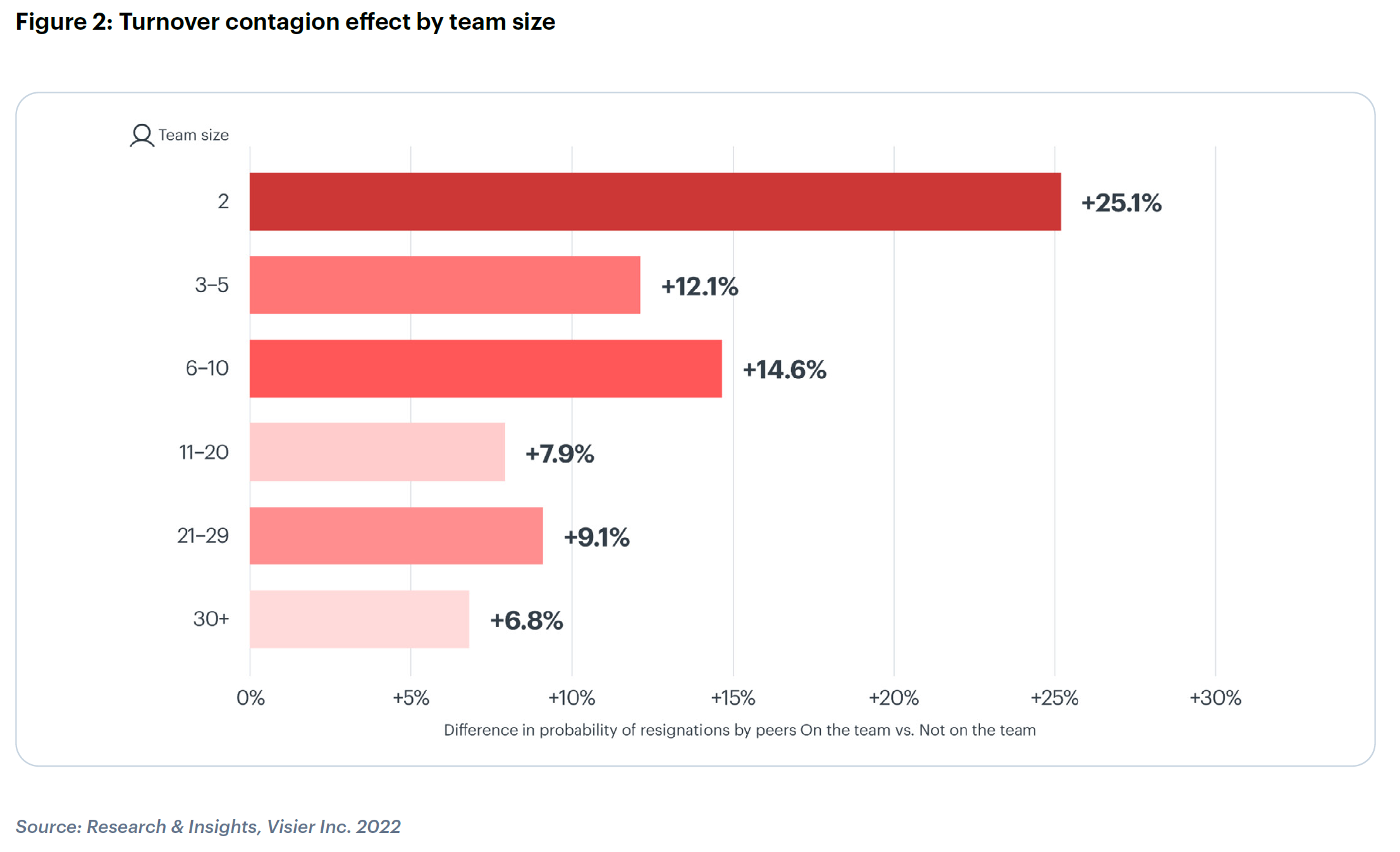
These results coincide with what is known about the link between team size and team cohesiveness and performance, whereby a commonly accepted benchmark for the ‘ideal’ team size lies between five to seven members. The fact that teams between six to ten members see an increased probability for turnover contagion effects seems to confirm this number, but not without pointing at a possible downside of team coherence; smaller, more interconnected teams might perform better but the risk of turnover contagion also increases.
Critical time frame for turnover contagion effects starts at around 45 days, and peaks at 70 days after the initial resignation. Knowing the time frame that defines turnover contagion helps to plan for an intervention. Mitigation strategies for turnover contagion should aim at 45 to 135 days after the first team member resigns.
A colleague quitting job can trigger such considerations even in employees who had not thought about this before. This is because among other reasons, people leave an organization in reaction to their team members resignations. Workforce planning and analysis that includes forecasting how many employees might leave, could add turnover contagion as additional risk factor as even small improvement in accuracy can lead to significant savings.
There are certain actions people managers and team leaders can take to try to prevent turnover behavior from turning into uncontrolled contagion effect. Predictive models are based on historical employee data and employee events, and calculate the probability based on the similarity to previous employees who quit the organization. There are various resignation attributes to include in an employee risk profile, for example:
- Job title and internal organization
- Age and tenure
- Location
- Compa-ratio
- Performance rating
- Time since last promotion
- Training hours
- Peer resignation events
Also, managers and team leaders can try to mitigate the risk of turnover contagion.
Watch out for employees’ pre-quitting behaviors such as decreased productivity, low commitment to long term timelines, or leaving the work early more frequently than usual are visible signs of disengagement and might reveal quitting intentions. Especially critical importance are the months after a team members resignation that have higher risk of subsequent leaves.
Be aware of the time span after a team member’s resignation as the risk of subsequent resignation is increased most for 135 days after the first team member’s resignation. During the first 4.5 month after losing a team member talent retention activities are particularly important. In that span focus on career conversations.
Strengthen social ties beyond the team. Especially in hybrid or remote work environments it has become increasingly important to enable social ties to others at the organization. One way to achieve it is cohort on boarding. Early connection beyond the immediate teams can potentially mitigate the risk of falling to turnover contagion.
Source: https://www.visier.com/lp/turnover-contagion/
How People Analytics Is Transforming the HR Landscape?
Firms are building their people analytics functions in different way. Team size, composition, and organization vary widely. Also, priorities for capability development and maturation differ significantly. Organizations’ people analytics maturity and capabilities are between basic reporting and advanced analytics, but most companies would agree that they have a long way to go.
McKinsey has developed classical four step model of maturity in people analytics and called it growth trajectory stairways with five steps. The best people analytics teams may take one step back every two steps up, but their trajectory are always upward.
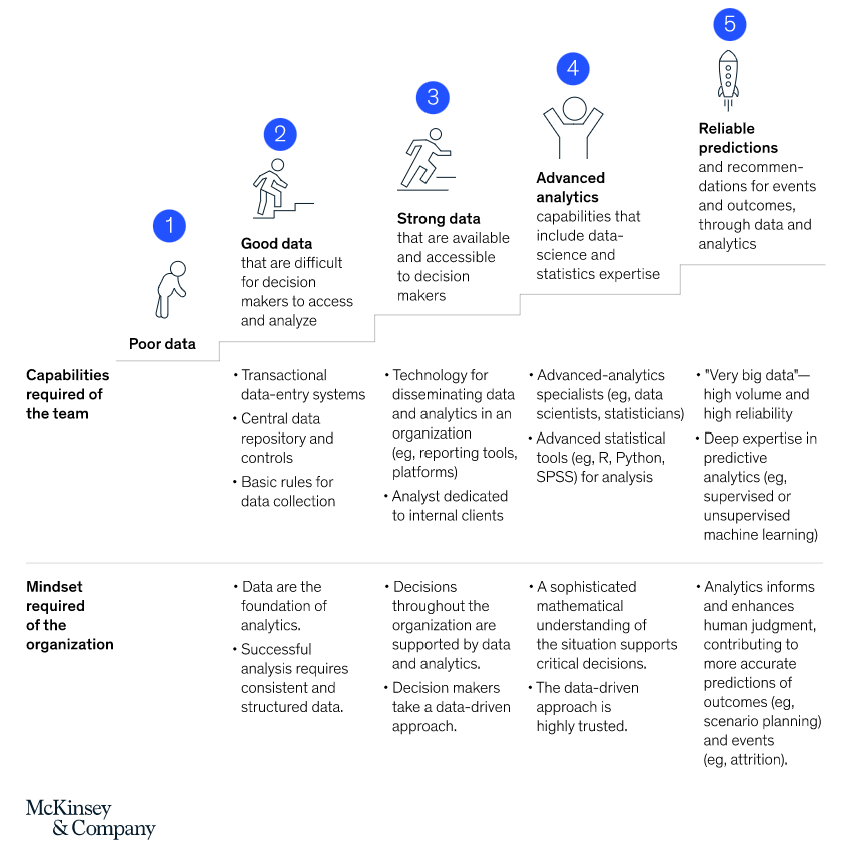
A foundation of high-quality data is a prerequisite to move from the first step – poor data – to the second step – good data. Usually, it means that data is extracted from core and transactional systems, then transformed (reshaped, cleaned, and re-coded) into more manageable structure that is loaded into data warehouse or similar data product. This is done, because the more time that analyst need to clean and understand data to make it usable even for simple analysis, the less efficient the people analytics is and the more time the team needs to develop their skills and capabilities. Significant resources, time, and investment are required to establish proper data management. This is iterative process with no definitive solutions. The process and its outcomes change as the internal and external environment shifts, systems are retired and renewed, and new links with stakeholders are established. As the operating environment changes, the technology used to manage and transform data need to be flexible. Taking an agile approach is fundamental in people analytics.
As soon as data foundation is in place, the people analytics should start making the useful data accessible to the organization and start experimenting with data democratization. Teams might focus on automating and visualizing HR dashboards via standard BI platforms such as PowerBI, Tableau, or Qlik. Other teams might prioritize custom build software using their own front-end developers. At some point organizations must make decisions whether to use homegrown platforms or third-party vendors.
Advanced analytics capabilities is the fourth step. Data scientists will use programming languages like R, or Python to join disparate sources of data, build models to help understand complex phenomena, and provide actionable recommendations to leaders making complex and strategic business decisions. The most advanced teams have created specific subspecialties in data science like natural-language processing, network analytics, or quantitative psychometrics. They increase their impact on their organizations by providing the advanced insights necessary to support strategic decision making.
Last step of the staircase is predictive analytics. We can dare to say that no people analytics teams are there yet. Reliable predictions will enable people analytics teams to analyse and explore practical options for management actions.
While some organizations have built fit-for-purpose predictive models—mostly for workforce planning—implementing predictive analytics in the context of employee selection, development, or engagement decisions requires a substantially scaled-up data-science operation, massive amounts of highly accurate data (“very big data”), cutting-edge algorithmic technology, and organizational comfort with how to address the impact on fairness and bias.
There are six best in class ingredients that help teams make impact, achieve success, and grow. These ingredients fall into three main categories. When you build people analytics from scratch this is what you should strive for.
Data and data management
- Significant and dedicated data-engineering resources
- Breadth and depth of data sources
Analytics capabilities
- Robust data-science function
- Strong translation capability
Operating models
- Innovation as the norm
- Clear alignment with clients and organizational use cases
Over time, as organizations become increasingly dependent on the quality of their insights, the best people analytics teams play a stronger role in shaping the HR agenda, influencing how the organization manages its talent at both a policy and a process level.
Below is set of questions that will be helpful to leaders who want to identify where their organization’s people analytics function is now and where it is heading:
- Where is the organization on the people analytics stairway? Where does it aspire to be in the next year, three years, and five years?
- How does the organizational context influence the mandate of the people analytics team?
- What ingredients does the organization possess today, and which does it need to build?
- How should the organization determine its priorities in building people analytics capabilities? For example, should it build to support certain specific internal use cases, or should it build a broad bench of capabilities to support an unpredictable or rapidly changing internal environment?
- If the organization had to get one thing right over the next 12 months, what would it be? What would get in the way of its getting there?
Predictive Retention Projects
We all are aware of growing talent shortages. Due to that fact, many companies express their interest in developing predictive models to anticipate and address employee attrition and look for ways to stay ahead of their competitors thanks to better talent retention.
Typical predictive retention project is looking to answer following questions:
- To what extent is my organization at risk for attrition within key segments of our workforce now and in the future?
- What are the factors that are influencing attrition/retention?
- How can I identify and prioritize interventions that could reduce the potential turnover within key workforce segments?
- How can I determine which interventions will pay off and how much will I save?
Organizations often are encountering challenges in designing predictive retention models. Such analysis should lead to business improvement so they require careful consideration of various factors.
These concerns fall into three overall categories.
Sense
- Use external market/industry trends, stakeholder insights, and voice of the employee to identify priority issues and opportunities to explore.
- Focus on data that is both actionable and relevant to addressing the problem, not just that which is easy to obtain.
- Secure stakeholder commitment from the top down.
Analyze
- Provide adequate time in the schedule for up-front data acquisition activities.
- Conduct a thorough data examination before moving on to modelling.
- Engage subject matter experts (SMEs) and stakeholders iteratively during the model development process.
- Maintain, refresh, and fine-tune models on an ongoing basis.
Act
- Develop stories to distil the key messages that drive executives’ engagement and eagerness to act is a critical success factor.
- Drive actions from business realities as well as the model output.
- Project investments and impacts of potential initiatives as an input to decision-making.
Organizations will often rely solely on engagement surveys or only use workforce data (such as demographics and compensation) that is easily accessed through a traditional human capital management platform. While these are fundamental inputs, the data that will truly provide insight often resides within other systems. For example, work-life balance and burnout insights can come from obtaining shift behavior and overtime patterns in labor scheduling systems, nights away from home from travel records, and after-hours activity from calendars. Quality and safety systems can indicate potential areas where employees may be under duress. Learning systems provide insights on development opportunities taken advantage of, and performance management and skills tracking systems can help to assess job fit. Further, including non-HR data, such as financial results, can help assess the impact of attrition and remediation on business performance.
Predictive retention is a tool to provide intelligence that can help manage the risk of turnover and associated costs. Yet, these types of projects require more than just understanding of mechanics of AI and ML. They require consideration of the context of the roles, the questions that need to be addressed, and the ability to translate the findings into actionable outcomes. If your organization is looking to undertake such efforts, consider the following questions:
- What roles or segments of the workforce do I want to focus on and why is retention important to the organization’s strategic direction?
- Do I have a clear set of hypotheses that are guiding my data collection and analysis?
- Have I identified the most relevant data and addressed the concerns of the owners of the data?
- Have I set out a process of cleaning and transforming my data once I have collected it?
- Do I have a process for engaging SMEs throughout the model design, development, and interpretation?
- Have you developed a clear change management strategy at the beginning of the project that addresses stakeholder management and communications to ensure follow through and actions?
Attrition analytics projects require diligent planning and grounding in a solid understanding of the market dynamics surrounding the organization, a thoughtful analytic process that takes advantage of the right data and expert involvement, and engaging communication of results that emphasize a quantification of the results projected from recommended actions.
Top 10 Most Demand Skills for the Next 10 Years
- Digital Literacy
- Data Literacy
- Critical Thinking
- Emotional Intelligence
- Creativity
- Collaboration
- Flexibility
- Leadership Skills
- Time Management
- Curiosity and Continuous Learning
Free E-book Alert!
Handbook of Regression Modelling in People Analytics by Keith McNulty
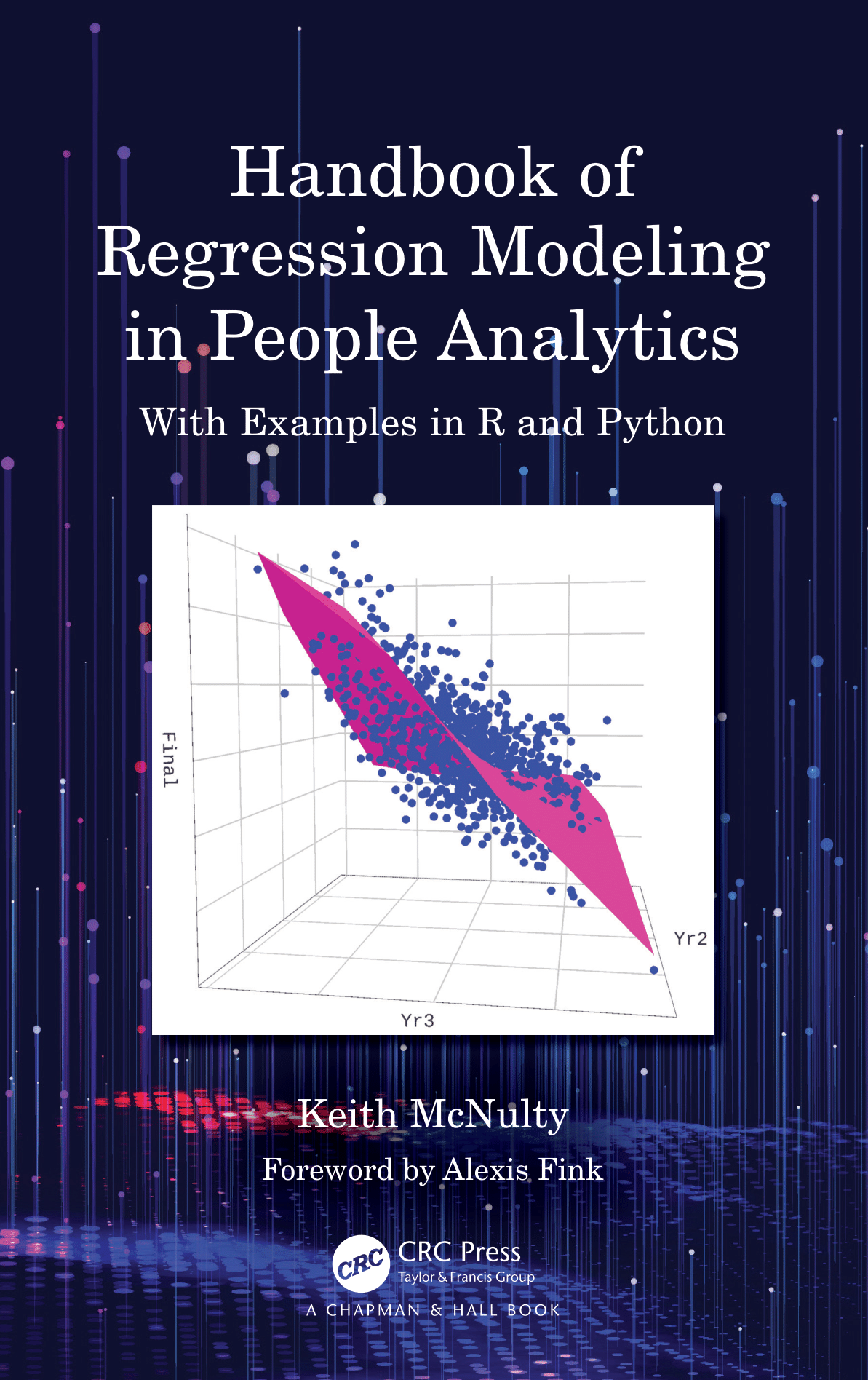
The book’s author describes it this way: “My motivation for writing this book, which is to give others—whether working in people analytics or otherwise—a starting point for a practical learning of regression methods, with the hope that they will see immediate applications to their work and take advantage of a much-underused toolkit that provides strong support for evidence-based practice.”
This book contains samples implementation in R, Python, and Julia. Also, particular datasets are available to practice implementation of regression methods in people analytics.
Link: https://peopleanalytics-regression-book.org/
Inspiring quote
“Failure is not an option.” – the tag line of the 1995 film Apollo 13
Want to be a Sponsor?
Would you like to be a sponsor of my newsletter? Contact me: sebastian@szachnowski.com.
Thank you for reading my newsletter! I’ll be delighted to get your feedback about the newsletter and this issue.
Do you know someone who might be interested in this newsletter? Share it with them.
You can also:
- Subscribe to my newsletter https://szachnowski.com/
- Connect with me on LinkedIn https://www.linkedin.com/in/sebastianszachnowski/
- Follow me on Twitter https://twitter.com/sszachnowski
- Follow me on Instagram https://www.instagram.com/sszachnowski/
- Send me an email sebastian@szachnowski.com